Bayesian A/B Testing: A Powerful Reasoning Model
A/B testing goes hand-in-hand with every marketer’s CRO strategy. Being a marketer or a CRO practitioner, you can’t underrate the value of embracing a culture of experimentation in your organization. Yet, finding an optimal variation to achieve higher conversions has remained a persistent challenge for online businesses throughout.
In its classical form, A/B testing operates as a binary model—a null hypothesis that needs to be rejected to accept the alternative hypothesis. Owing to the complicated process of deriving insights from an experiment and taking crucial business decisions based on them, CRO practitioners have been adopting the more credible and intuitive model over the classical Frequentist one. It’s the Bayesian model.
Download Free: A/B Testing Guide
The Bayesian model proves the evidence of the reasoning behind an experiment you run. In this blog post, we have explored the Bayesian model in detail, compared it with the classic Frequentist approach, and discussed its use cases.
What is the Bayesian approach?
Bayesian reasoning is fundamentally a belief-based approach with its foundation in Bayes’ theorem. The theorem presents a mathematical framework to update your existing beliefs with the influx of new information.
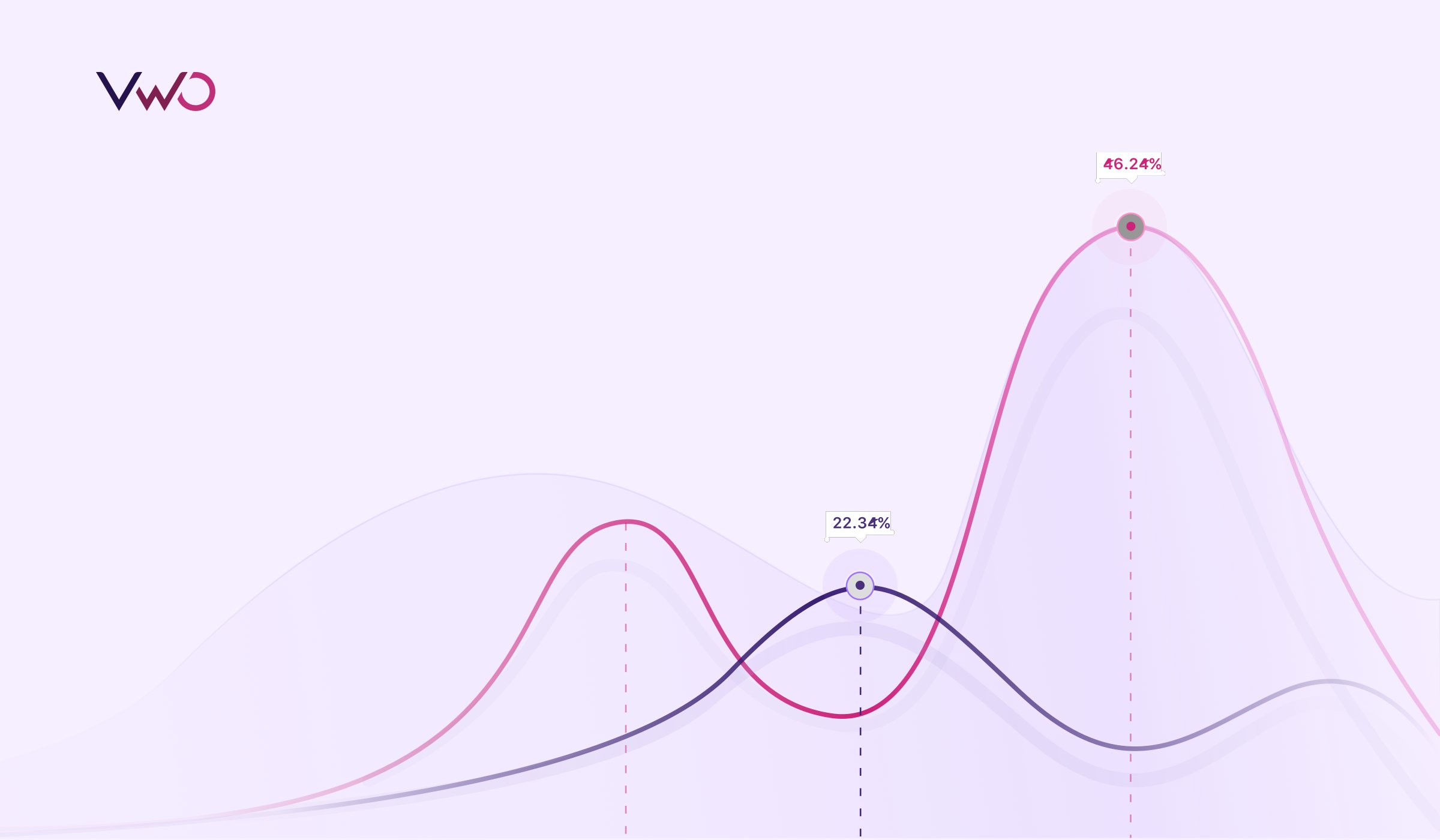
Here is an example for you. A doctor can diagnose a medical problem in a patient following either one of the approaches. As a Frequentist, he would have a fixed model set up against a patient’s specific symptoms for his diagnosis. He may probe the patient for them and identify the cause based on the fixed model he has in place.
Contrarily, as a Bayesian, the doctor would still have a model. He would probe the patient for gauging his condition and identifying symptoms, and in addition, he would like to know the history of any past pains in the patient. Hence, his diagnosis will include the current symptoms and historical symptoms in identifying the actual cause. This approach will update his existing model with new information, which can lead to faster innovation with no extra cost, time, or energy spent in the process of diagnosis.
An example from StackExchange makes this concept even simpler.
Say you’ve misplaced your phone, and since this happens all the time, you have got yourself a phone locator. Your phone has an instrument attached to its base, which signals it to start beeping when you press a button on the phone locator. The problem is, from where should you begin your search in the house?
If you are a Frequentist, your built-in model would be identifying the area based on the direction of the sound of beeps. Thus, you will run after the beeps to find the device’s location in the house.
Yet, as a Bayesian, you would recall the locations where you found it the last time it went missing. Did you find it on the kitchen slab or buried under the laundry? You will have reasons behind putting the phone in those places. Apart from the sound of the beeps, recalling this information will act as a prior that can assist you in making an evidence-based decision. In this case, it will figure out where to begin the search.
The critical aspect of Bayesian thinking is that it enables you to explore the pre-existing beliefs (priors) during your research, and these beliefs get updated with evidence data resulting in new beliefs (posteriors).
In the context of A/B testing, why is the Bayesian approach more beneficial than Frequentist?
You can hear a Frequentist, who does not like to talk about one-time events, murmuring—”Did I arrive at the truth correctly?”. On the contrary, a Bayesian, who cares more about updating opinion based on data than finding the ultimate truth, can be heard out loud declaring—”I don’t know what the truth is, but I believe my initial opinion would change now since I have a new piece of information with me!” Pretty confident? Well, yes!
We ran an A/B test using VWO to check if a banner appearing on the exit intent of visitors can increase lead generation on our blog. Looking at it through the Bayesian lens, we see a varying degree of confidence in all possible conversion rates in the graphical representation. We concluded that control (no banner) is the winner, and also, there has been an overlap in the conversion rate between 0.25% and 0.6%.
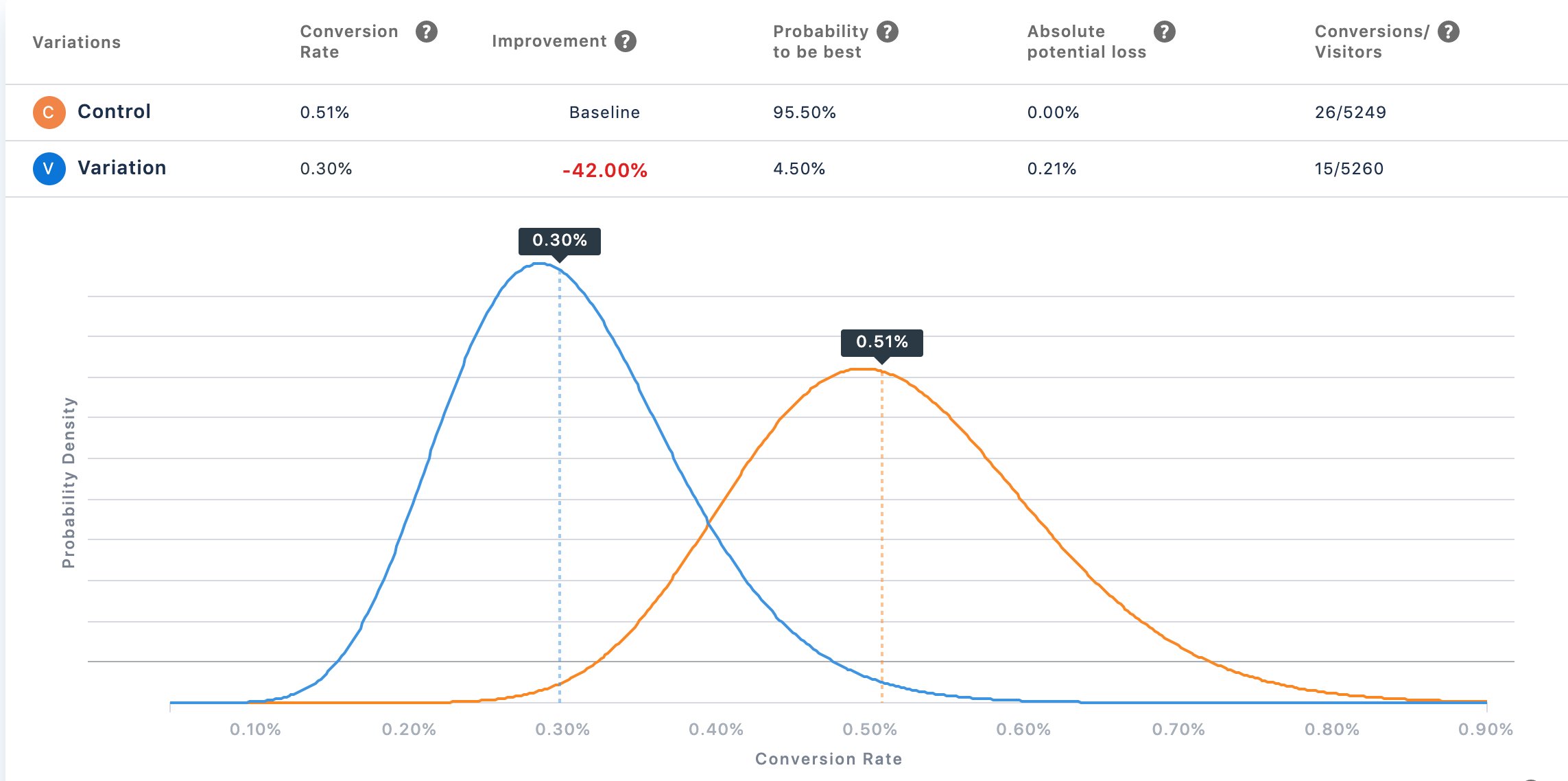
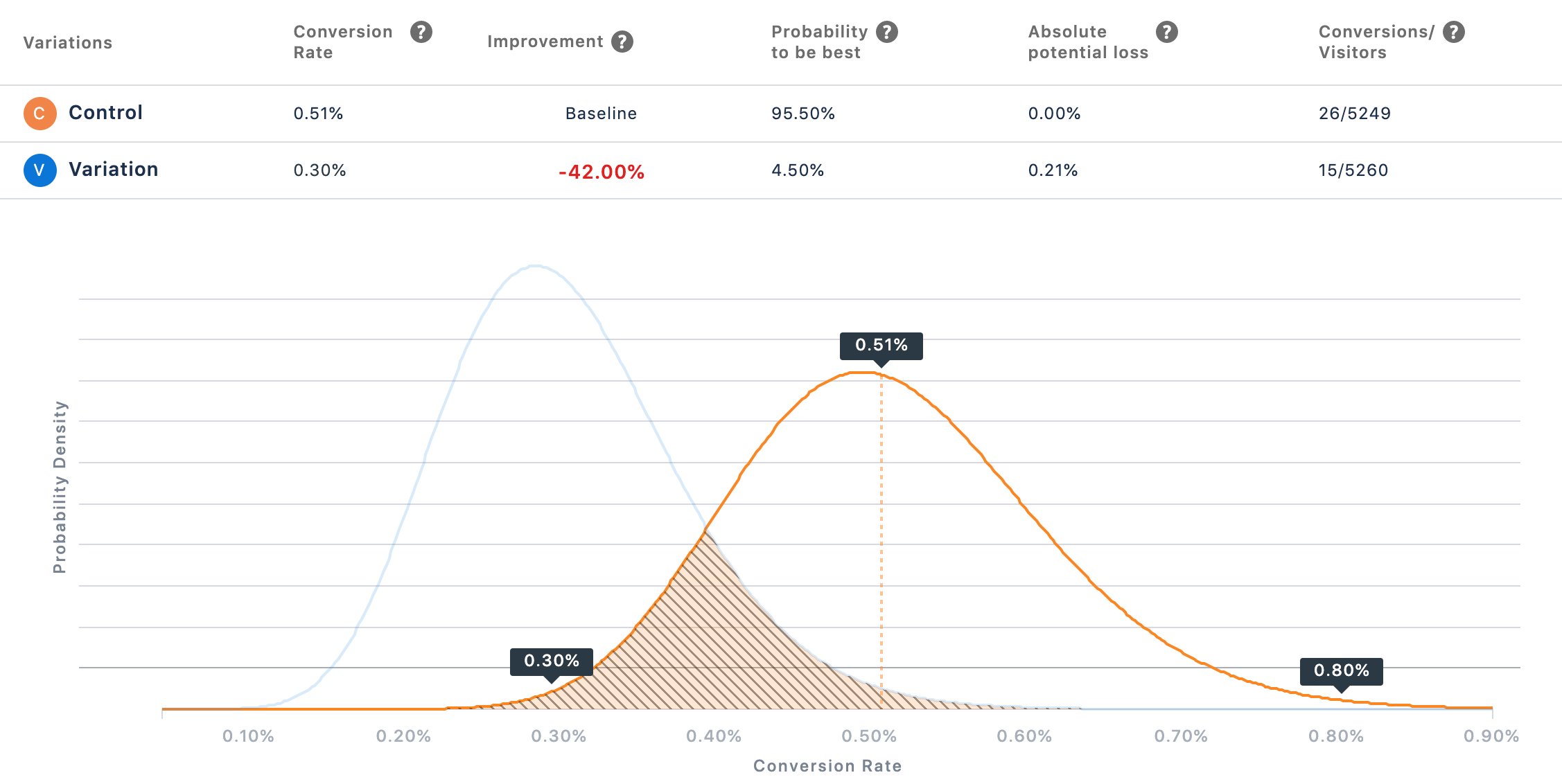
On the other hand, the Frequentist approach returned a p=0.042, also concluding that the control is the winner.
The Frequentist approach is steadfast in reaching a significant number of visitors to return a p-value, which is hard to put in a business context. What can you discern with a p=0.042 here? It’s likely to leave you confused.
As a marketer or a growth leader, you would want your tool to do the heavy-lifting when it comes to statistics and give you results that help you make good business decisions.
Bayesian provides a more sensible and intuitive way to optimize your CRO efforts. It updates your opinions backed by evidence when you deduce your prediction and induce the learning in the experiment cycle with a knowledge update, as shown in the image below.
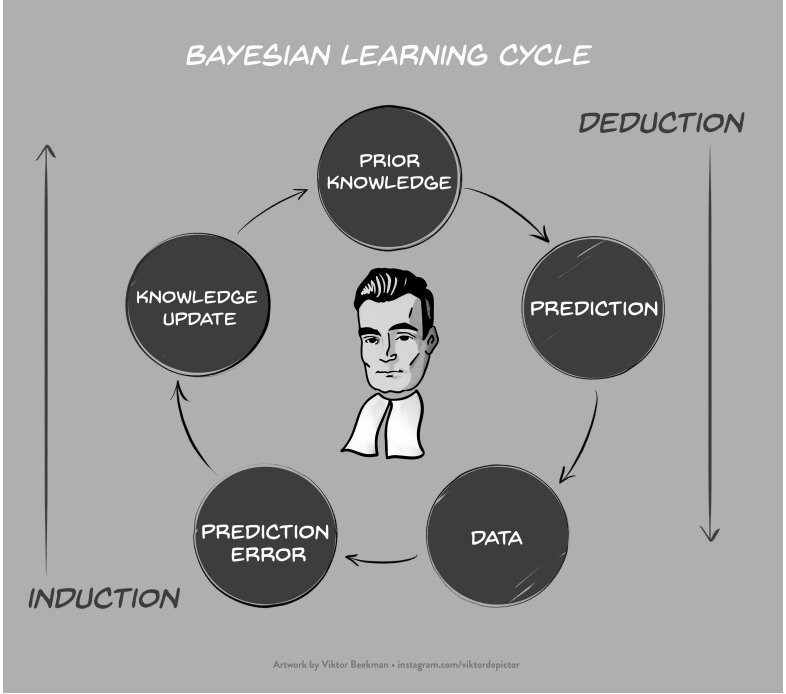
VWO SmartStats—the Bayesian way
VWO goes the Bayesian way with SmartStats, a Bayesian-powered statistics engine for A/B testing. This engine gives you intelligent results to make smarter business decisions and reduces your testing time.
The Bayesian approach enables you to incorporate knowledge into your experiments iteratively. SmartStats catalyzes this approach using a non-informative prior where all conversion rate possibilities are equally likely. In addition, it ensures that you stay in control and can monitor the test as it progresses and reaches its significance over a period of time before it concludes.

Let’s go back to the exit-intent banner example discussed in the blog. For the test, we observed that the distributions were wider initially. However, as shown below, you can figure out that they started to shrink with more data.
The probability to beat baseline during the start of the test was close to 50%, but as the test progressed, it reached 95% confidence after reaching 1000 visitors, declaring the control as the winner.
If you look at the progress of this experiment with respect to time and traffic, it looked like this with the lowest number of visitors, showing variation leading (non-significantly):
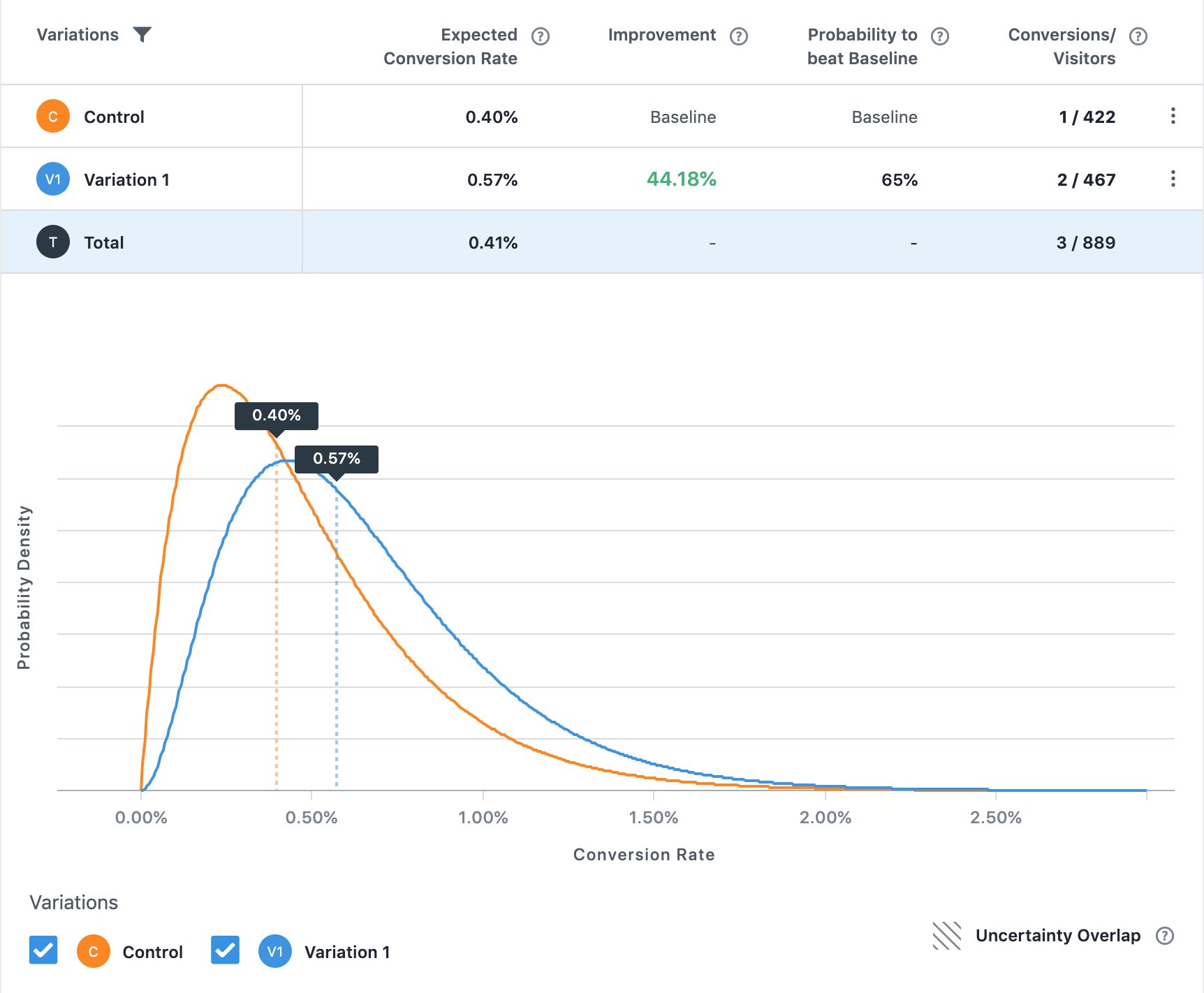
And, this with ~1000 visitors, variation dropping steeply and control expanding:
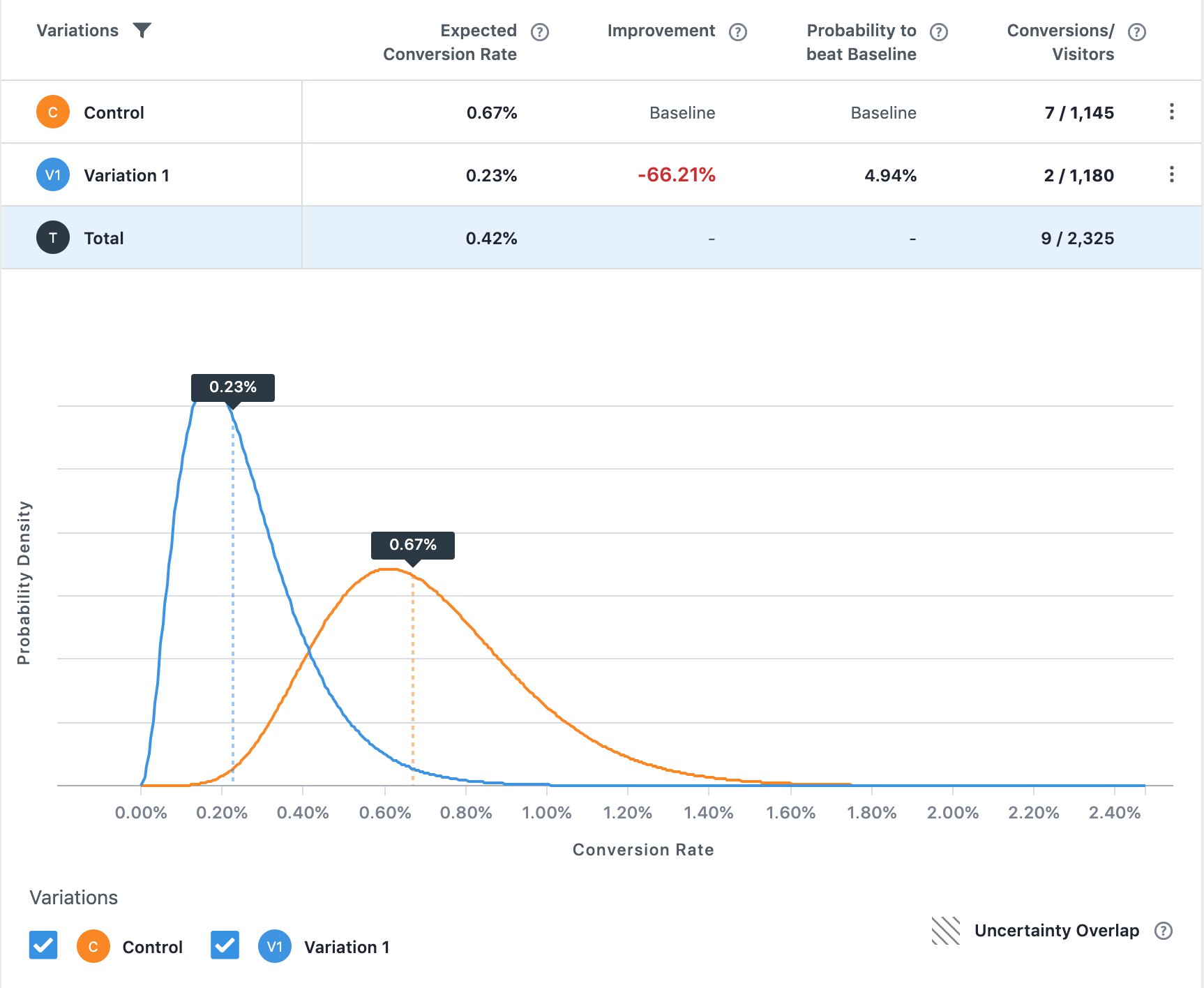
The final graph declaring control as the winner:
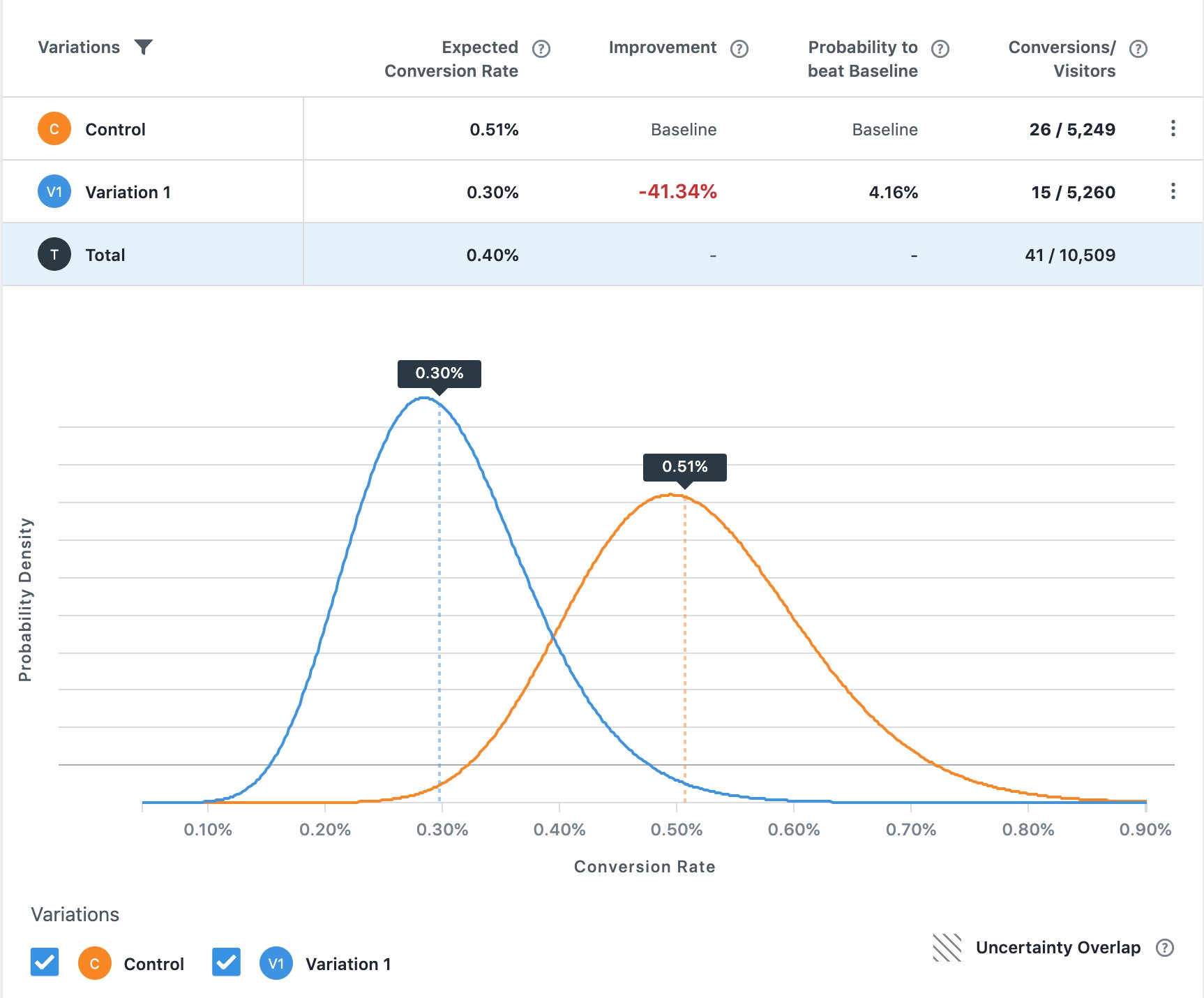
Download Free: A/B Testing Guide
How does Bayesian A/B testing allow faster innovation?
With the ability to incorporate belief as part of the experiment, the Bayesian approach enables you to make faster decisions with lower experimentation costs as compared to a Frequentist approach.
It is common to use a Bayesian approach where running an experiment is costlier, and you don’t have enough data to make a decision, whether it is a medical diagnostics test to discern the probability of having cancer or the email being spam.
Also, the Bayesian approach allows you to feed a posterior of one experiment as prior to another. Therefore, an A/B testing tool based on this model enables you to consistently and quickly optimize your experiments for conversions. You do not have to learn new data from your experiment every single time, instead, feed the posterior (read: update) to a prior, iteratively, to significantly determine improvement with less data.
With the exit-intent banner experiment, extensively discussed in the blog, we took a quick decision looking at the progress of the test as soon as it reached its significance. We did so to mitigate the loss of the opportunity metric that was MQLs in this case. The test was concluded with an observation that an exit-intent banner doesn’t work on our blog. This observation can act as data-backed evidence (posterior) to be fed as a prior to our subsequent experiments around blogs.
So don’t wait. Take a plunge, fail fast, learn effectively, and grow exponentially with iterative A/B testing that promises opportunities for innovation in your business to witness astronomical conversions.
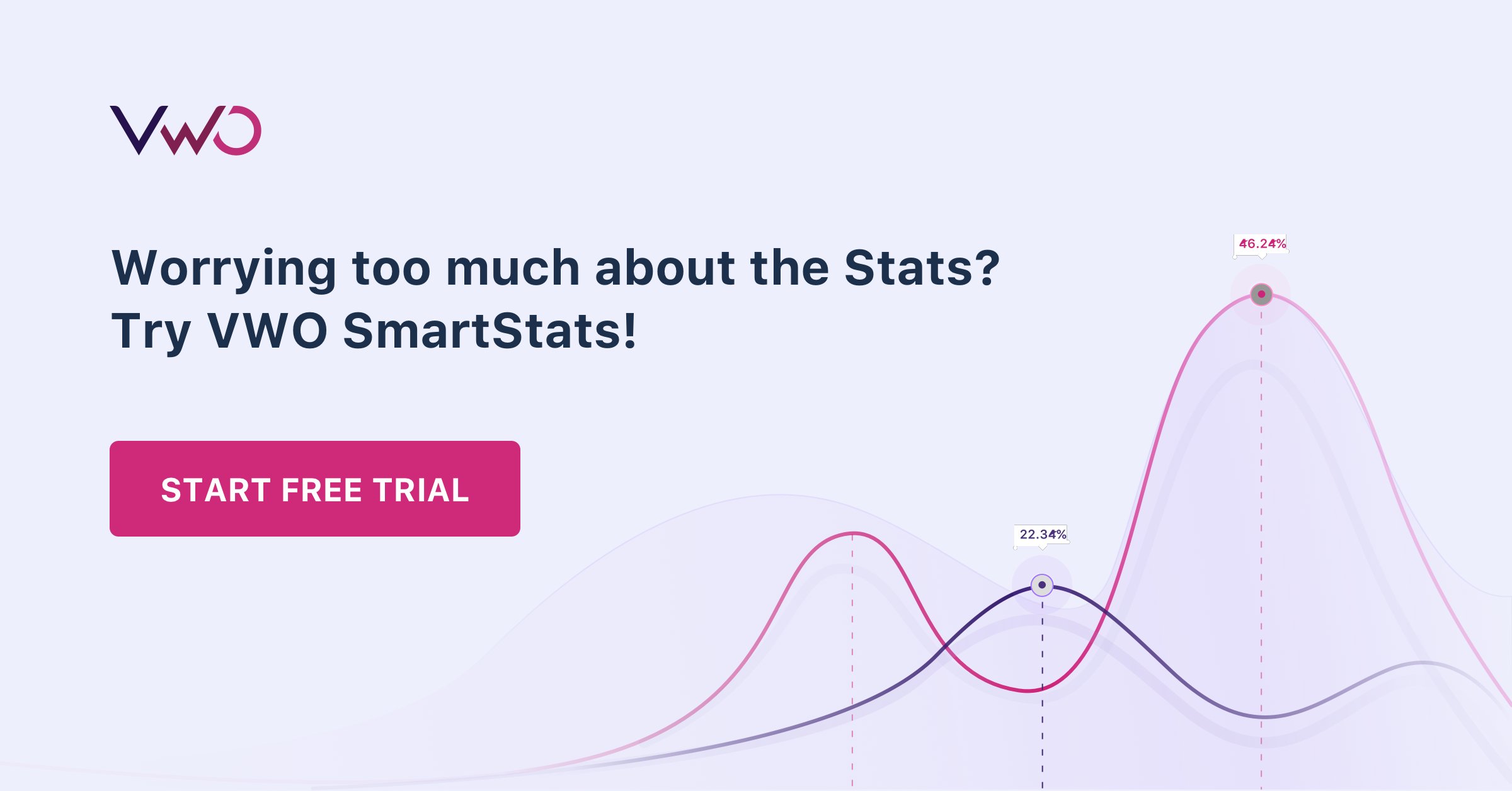